Artificial intelligence shows promise and pitfalls, experts say
AI can be a benefit in cardiovascular care, but a responsible approach is needed
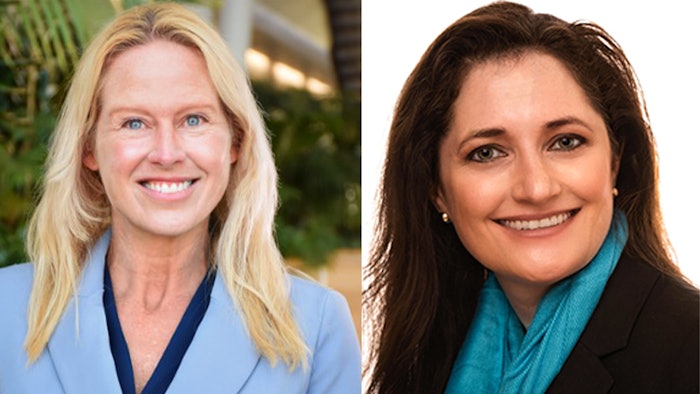
Artificial intelligence is making its way into more aspects of medical care — and cardiovascular care is no exception.
But while the full potential of this technology has yet to be realized, its use should be approached with caution and care, said Randi Foraker, PhD, MA, FAHA, FAMIA, director of the Center for Population Health Informatics at the Washington University Institute for Informatics in St. Louis, Missouri.
Dr. Foraker, who along with Jessica L. Mega, MD, MPH, cardiologist at Stanford Health Care in Palo Alto, California, will lead Monday’s session, AI in the Clinic and Lab: Promise, Pitfalls, and the Data Police.
One of the main uses for AI in cardiovascular care is in imaging, Dr. Foraker said.
“AI methods are really designed to pick out patterns and abnormalities in those patterns,” she said. “That’s why it’s ideal for images, because normal images look alike and are very predictable in that look, but abnormalities in images are easy to ascertain through AI. The subtleties in those abnormalities are detectable. Those could be blood vessels of the heart or areas in the brain during a stroke.”
In addition to imaging, AI has been evaluated for numerous other areas, Dr. Mega said.
“AI in cardiology is being discussed in a number of broad settings, including the diagnosis, treatment and prevention of disease,” she said. “Administrative AI tools are also working their way into clinical practice through technologies, such as voice recognition software and health care claims processing.”
Artificial intelligence could be an important tool for cardiovascular care because it can spot patterns a human eye might miss, Dr. Mega said.
“AI can be used to detect signals or patterns that are not easily recognizable on the surface,” she said. “This type of anomaly detection can be used in different ways, from identifying particular populations of people who are being undertreated to finding cases of medical overtreatment.”
Dr. Foraker said AI has the potential to dig deeper into data and uncover biases that otherwise might be overlooked. However, she cautioned that it must be used in the right way.
“It’s going to be very difficult to uncover biases with AI if we aren’t looking for them, if we aren’t anticipating that the data we are using may be biased in some way,” Dr. Foraker said.
One example of this is using a machine learning algorithm to evaluate data on 30-day readmission rates for heart failure during the height of the COVID-19 pandemic. Dr. Foraker said it is important to make sure the algorithm understands underlying factors that were happening during that time.
“We may not see the high of readmissions for heart failure (during that time) that we might anticipate because the population mix changed during COVID,” she said. “We started seeing and prioritizing COVID patients over other types of diagnoses and symptoms.
“If we’re not thinking about those potential biases and the potential selection bias of patients, then artificial intelligence wouldn’t tell us about the bias. We have to be on the lookout for it, and we have to build it into our model training and retraining.”
While AI may be useful in many areas, Dr. Foraker said it is far from perfect — it is only as good as the data being fed into it.
“One potential pitfall of AI is to assume that AI is the magic bullet for evaluating data, and in particular evaluating the often-imperfect data we have at our disposal,” she said. “So no matter how fancy or advanced it is, it’s not going to fix the problems in the underlying data source.”
Dr. Mega agreed, adding that it is crucial to understand the source of the data being used to create the AI models and to assess whether it is representative of the population being treated.
“Using incomplete data and/or data that includes biases based on particular values could lead to inaccurate assessments,” Dr. Mega said. “The application of AI in health care requires broad testing and engagement of many people, with open feedback loops and a focus on quality.”